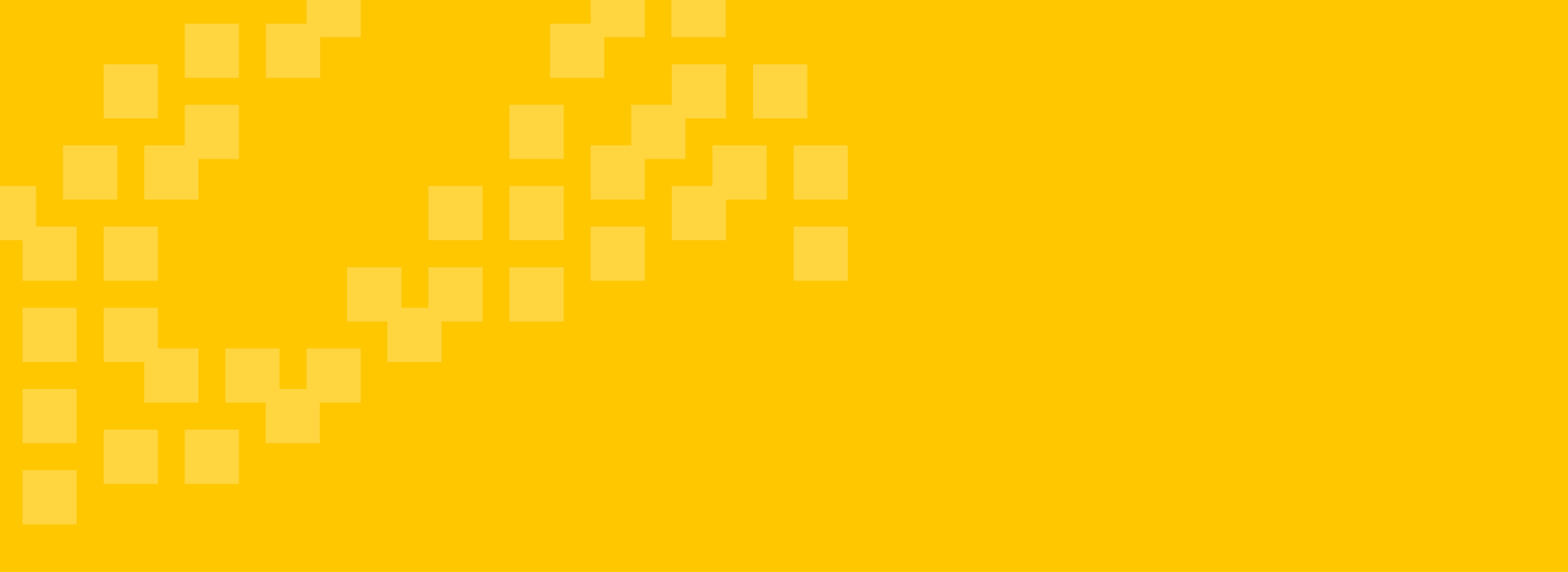
Model Deployment
Bringing AI Systems to Life in the World
After models are trained--drawing on refined hardware, expansive datasets, and intensive computation--artificial intelligence systems are deployed into real-world contexts. This is the phase where AI begins to interact with society: powering recommendations, managing infrastructure, assisting diagnostics, automating decisions, or interpreting images and speech. Model deployment makes AI visible and operational, shaping the systems and environments people live within.
Deployment is not simply the endpoint of technical development, but a process of integration, governance, and impact. It determines not only how models are used, but who uses them, on what terms, and with what consequences.
-
AI deployment involves several interlocking steps that prepare a model for real-world use:
Data Center Operations
Once models are trained, they are hosted and run on vast networks of cloud infrastructure. These data centers--operated by companies like Amazon Web Services (AWS), Google Cloud, and Microsoft Azure--require massive energy inputs for computing and cooling, as well as large volumes of water for thermal management. Their location is often driven by access to cheap land, energy, and favorable tax incentives, rather than local needs or sustainability.Model Integration and Application Design
AI models--ranging from vision systems to language models–must be integrated into applications or services. This involves backend engineering, user interface development, and operational scaling. At this stage, decisions about accessibility, accountability, and user control are often made without community input, especially in tools deployed across education, healthcare, and public governance.Content Moderation, Feedback Loops, and Fine-Tuning
Even after deployment, models require ongoing monitoring and refinement. This work is frequently outsourced to a global workforce of data workers–data annotators, content moderators, and task workers–many of whom operate under contract through platforms like Scale AI, Appen, or Sama. Their labor is crucial to model accuracy, yet remains invisible and undercompensated. -
Model deployment is often where the perceived promises of AI—efficiency, insight, accessibility—are put into practice, but it is also where many of its underlying asymmetries and tensions become most visible. While this stage is frequently framed as less resource-intensive than training, the cumulative energy and infrastructure demands of running models at scale—especially in widely used applications—can be significant. Inference workloads for large language models, for example, may consume substantial amounts of electricity, particularly when accessed by millions of users in real time. Over time, this persistent computational load can rival or even exceed that of initial training, especially when models are embedded in always-on services like chat assistants or translation engines.
These systems are hosted in data centers whose environmental impact depends heavily on their location and energy sourcing. In some regions, data centers continue to rely on fossil-fuel-dominated grids or are situated in areas facing water scarcity, where evaporative cooling adds pressure to already strained ecosystems. While many cloud providers have announced carbon neutrality goals and investments in renewables, independent verification and granularity of reporting remain limited, making it difficult to assess the actual progress toward sustainable deployment.
Meanwhile, the global labor system that keeps deployed models functioning is shaped by steep inequities. Content moderators in Kenya, for instance, have worked under traumatic conditions to label violent or abusive material for generative AI tools, often without access to psychological support or fair pay. Elsewhere, platform workers labeling medical or legal data lack recognition and job security, despite contributing to high-stakes applications.
Deployment is also the stage where AI systems begin to interact with social institutions and governance processes, often in ways that raise important questions about accountability, transparency, and public oversight. In areas such as law enforcement, social welfare, education, and healthcare, algorithmic tools are increasingly used to support decision-making. While these systems are often introduced with the aim of improving efficiency or consistency, their impacts can vary significantly depending on context, design, and implementation.
In some instances, predictive policing tools have been associated with reinforcing existing patterns of surveillance in communities already subject to high levels of monitoring. In welfare systems, automated scoring mechanisms can introduce opacity into decisions about eligibility or support, making it difficult for individuals to understand or contest outcomes. Similarly, when language models are integrated into public-facing applications—such as tutoring platforms or health advice tools—they may inadvertently reproduce bias or inaccuracies from their training data, particularly when used at scale.
These outcomes often reflect the cumulative effect of institutional priorities, limited participatory design, and broader structural dynamics. Recognizing deployment as a site of governance—not just technical rollout—can help ensure that the systems we adopt are better aligned with the diverse needs and values of the communities they affect.
-
Climate and ecological breakdown → AI model deployment relies on a growing network of energy- and water-intensive data centers. In places like the Netherlands, Chile, and South Africa, local communities have voiced concern over land and water use by foreign-owned tech infrastructure. Data centers may compete with residents for water in drought-prone areas or contribute to rising emissions in fossil-fuel-powered grids. The carbon footprint of model deployment continues to grow as AI applications scale across sectors. Inference—the process of running models after training—has become a major source of emissions, particularly for multimodal models or real-time applications (like surveillance or translation). These emissions are difficult to track due to limited transparency from major providers.
Infrastructural burden → Infrastructurally, the energy and resource demands of continuous inference are not borne equally. While users in high-consumption regions benefit from responsive, AI-enhanced services, the power required to sustain those services is often generated or hosted in locations where environmental protections are weaker, or where water and energy resources are already under pressure. These costs are frequently absorbed by communities that had little say in whether or how such infrastructure would be installed.
Social and procedural vulnerability → Socially, many of the risks associated with deployment fall on individuals with the least capacity to shape or resist them. People navigating immigration systems, applying for social benefits, or undergoing background checks may find themselves assessed by AI systems they neither see nor understand. Decisions are increasingly mediated by technical systems whose logic is inaccessible and whose accountability remains diffuse. For these individuals, being subject to AI is not a matter of adoption or choice—it is something that happens to them, often without recourse.
Invisible labor → At the same time, the human labor required to sustain deployed models—through moderation, feedback, and exception handling—continues to be outsourced to workers whose contributions are essential yet undervalued. These workers operate in content moderation hubs, data centers, or service subcontracting firms, often far from the sites where AI’s value is captured and celebrated. The distribution of this labor follows global patterns of economic disparity and institutional opacity.
Epistemic consequences → Finally, there are subtler forms of burden that are harder to quantify but no less significant. As AI systems shape information flows, institutional routines, and cultural narratives, they influence whose knowledge counts, whose experiences are legible, and whose priorities are encoded into the infrastructures that increasingly govern daily life. For many communities, the cost of deployment is not just environmental or procedural—it is epistemic: a slow erosion of space to contest, reinterpret, or opt out of dominant systems of logic and control.
Understanding who bears the burden of AI deployment thus requires more than tracing emissions or quantifying error rates. It requires asking who is being asked to adapt, accommodate, or absorb the consequences of technological decisions made elsewhere—and what forms of justice or redress are available when the burdens become too heavy to bear.
-
Model deployment relies heavily on a small group of hyperscale cloud providers—such as Amazon Web Services, Microsoft Azure, Google Cloud, Alibaba Cloud, and Tencent Cloud—which provide much of the infrastructure through which AI models are hosted, maintained, and accessed. These actors do not merely offer storage or computing services; their infrastructure effectively shapes the conditions of deployment, influencing where models can be run, how quickly they can scale, and under what technical and contractual terms. Their market dominance gives them considerable influence over the pace and direction of AI innovation, as well as the standards and practices that govern it.
Many of these companies are headquartered in, and operate under, the regulatory frameworks of countries such as the United States and China, which indirectly extends their geopolitical influence into digital infrastructures worldwide (though, as Michael Kwet notes in Digital Degrowth: Technology in the Age of Survival, prevailing narratives that frame China as an equal technological superpower to the United States may obscure the deeper structural asymmetries between the two, particularly in terms of platform control, standard-setting power, and global reach). This dynamic can affect how questions of data sovereignty, privacy, and compliance are interpreted and enforced—often aligning with the legal and commercial interests of powerful states. Meanwhile, smaller or lower-income nations negotiating infrastructure agreements may face constrained options, entering into partnerships with limited leverage or under conditions that reinforce technological dependence.
Initiatives to reclaim a degree of autonomy—such as the European Union’s push for digital sovereignty, or regional data center strategies in Africa and Latin America—signal growing recognition of the need for more context-sensitive and locally governed AI infrastructure. Yet in practice, these efforts are often hampered by uneven access to capital, talent, and trusted open-source alternatives. Without coordinated shifts in procurement practices, public investment, and governance models that support decentralization, model deployment is likely to remain a highly centralized process—with far-reaching implications not only for innovation, but for environmental sustainability, institutional independence, and global equity.
-
A planetary justice approach invites us to consider model deployment not merely as the endpoint of AI development, but as a phase of ongoing negotiation—where technical, ecological, and political decisions converge in ways that shape how AI is experienced on the ground. This means evaluating deployment not only by performance metrics, but by asking: Who is affected? How are harms addressed? Whose priorities and ways of knowing are reflected—and whose are sidelined?
Accountability in deployment must extend beyond algorithmic fairness to include the conditions that make AI operational. The labor of annotators, moderators, technicians, and infrastructure maintainers—often marginalized or made invisible—is central to keeping AI systems functional. Recognizing these individuals as co-creators of technological systems, rather than peripheral support, calls for improved protections, transparency, and opportunities for shared governance in the AI ecosystem.
A planetary lens also urges us to consider how deployment affects not only human communities, but the ecological systems and more-than-human worlds that sustain them. The siting of data centers, the extraction of water for cooling, and the generation of heat and emissions during model use all impact landscapes, species, and local ecologies—often without being acknowledged in AI governance discussions. Questions of justice here must include those beings and systems that cannot speak for themselves, but are nonetheless deeply affected by infrastructural expansion. This means shifting from metrics of efficiency toward ethics of interdependence, care, and ecological reciprocity.
Deployment processes should also be grounded in place-based governance. Communities hosting AI infrastructure—whether human or more-than-human—should have a meaningful role in shaping decisions about land use, water access, and energy flows. Benefit-sharing frameworks and impact assessments should account not only for carbon or costs, but for long-term ecological wellbeing, and the needs of future generations—both human and nonhuman.
Crucially, a planetary justice lens does not assume that deployment is always justified. It prompts deeper questions: Who are these systems for? What forms of knowledge or care are displaced? What would restraint look like in a just AI future? In some cases, co-designed alternatives, slower rollouts, or even choosing not to deploy may better align with collective well-being and planetary health than unchecked expansion.