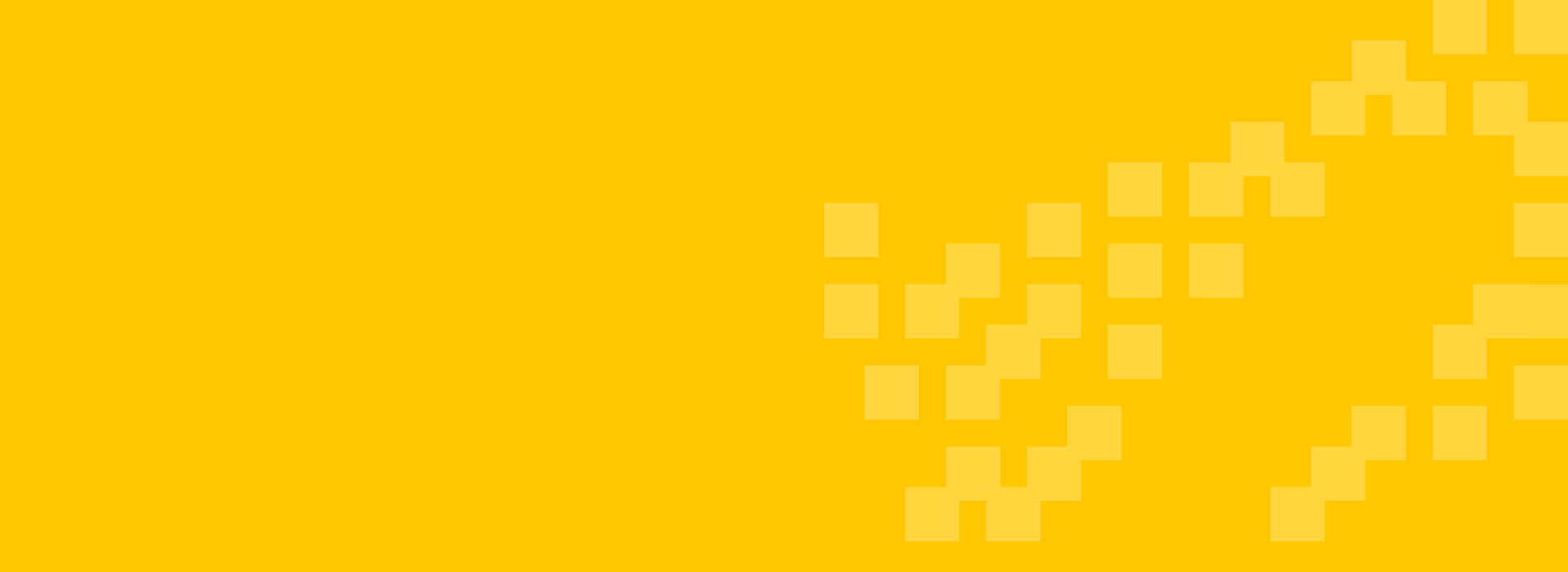
Equipment Manufacturing
Assembling the Physical Core of AI
While AI is often imagined as weightless software or invisible code, it runs on physical machines: servers, sensors, smartphones, data centers, and high-performance computing systems. These devices are the result of a globally distributed manufacturing ecosystem that brings together thousands of components--semiconductors, memory chips, power converters, hard drives--each built through labor- and resource-intensive processes. Equipment manufacturing turns refined materials into the physical backbone of artificial intelligence.
-
The production of AI hardware is vast and multi-tiered. It begins with the fabrication of core components, continues through global assembly networks, and ends with integration into AI-ready systems.
Semiconductor Fabrication
The semiconductor is arguably the most critical element of AI systems, enabling computation, storage, and networking. Producing these chips is one of the most complex industrial processes on Earth, requiring cleanrooms, hundreds of chemical and mechanical steps, and extremely pure materials. Most chips are made by a few foundries, such as TSMC in Taiwan,Samsung in South Korea, and Intel in the U.S., concentrating both technical knowledge and geopolitical risk.Component Assembly
Chips are then combined with motherboards, batteries, cooling systems, and other components in specialized facilities, often in China, Vietnam, Malaysia, or Mexico. These facilities source components from dozens of suppliers, forming a dense and opaque web of subcontractors.System Integration and Testing
Final assembly takes place in tech hubs like Shenzhen, Chennai, or Guadalajara, where AI-specific hardware--such as GPUs for data centers or edge devices like smart cameras--is tested, validated, and packaged for deployment. These hubs play a central role in preparing AI systems for real-world use but often operate under high pressure, with tight production cycles and minimal regulatory oversight. -
Although equipment manufacturing may appear more technologically advanced or cleaner than extraction and refining, it embeds similar patterns of inequality and risk. The environmental footprint of production is significant—especially considering energy and water use in semiconductor fabrication, the generation of electronic waste, and reliance on global logistics networks.
Socially, this stage of the supply chain depends on low-cost labor and infrastructure in export-processing zones and special economic areas, often benefiting from tax exemptions and weakened labor protections. Communities surrounding large factories may face pollution from industrial runoff, while workers endure repetitive strain injuries, exposure to solvents, and long hours without strong union representation.
Moreover, the design and assembly of AI infrastructure is shaped by decisions made far from where the work takes place. Workers in Malaysia or Vietnam may assemble devices based on design specifications from Silicon Valley or Seoul, yet rarely have input into broader questions about what these technologies do, or who they serve.
-
The burdens of equipment manufacturing are distributed across industrial and economic peripheries--especially in the Majority World--where infrastructure is cheap, labor is abundant, and regulatory environments are flexible.
Ecological breakdown → Semiconductor fabs and assembly plants consume vast amounts of energy and water. For example, fabs in Taiwan consume more water daily than some small cities, intensifying local water stress during droughts. Wastewater from chip cleaning and metal plating processes, if untreated, can carry heavy metals and solvents into surrounding ecosystems.
Climate breakdown → The global logistics systems that support equipment manufacturing--from transoceanic shipping of components to just-in-time supply chains--contribute significantly to emissions. AI-specific hardware is often shipped across multiple continents before deployment, multiplying its carbon footprint long before a model is trained.
Labor injustices → Manufacturing facilities rely heavily on outsourced and precarious labor. In places like Malaysia, Mexico, and the Philippines, reports have documented long shifts, low pay, and repetitive motion injuries in electronics assembly. Workers may be exposed to harmful chemicals such as trichloroethylene and isopropyl alcohol without adequate protection, and are often barred from organizing.
Resource colonialism → While AI devices are built in one part of the world, their design, intellectual property, and profits are concentrated elsewhere. High-value activities like design and systems engineering tend to occur in countries like the U.S., Germany, or South Korea, while the environmental and labor costs are externalized to manufacturing hubs in the Global South. In the end, this continues a pattern of development in which peripheral regions support innovation they have limited power to shape.
-
Equipment manufacturing, like other supply chain stages, is geographically concentrated in a few key regions--especially East and Southeast Asia, where governments have built powerful industrial policies around electronics manufacturing. Countries like China, Vietnam, and Malaysia are central to global assembly networks, while semiconductor fabrication is dominated by Taiwan (TSMC), South Korea (Samsung), and the United States (Intel, GlobalFoundries). Companies like NVIDIA--though not directly involved in refining or fabricating chips--exert significant influence over the materials supply chain through their hardware designs. Their demand for ever-more powerful GPUs--central to AI training--drives upstream pressure on foundries and component suppliers to source increasingly specialized and mineral-intensive inputs.
This concentration raises vulnerabilities to disruption--from pandemics and political tensions to natural disasters or export restrictions. For instance, U.S.-China trade tensions and export controls on advanced chips have intensified geopolitical competition over AI hardware, prompting efforts in the U.S., EU, and Japan to “reshore” production and reduce reliance on any single supplier.
However, shifting production away from current hubs poses environmental, labor, and economic trade-offs. New fabs require enormous investments in land, water, and energy; relocating them to the Global North may reduce one type of dependency while increasing others. Moreover, simply moving manufacturing sites does not guarantee more equitable or sustainable practices if global supply chains remain extractive in nature.
-
Addressing the justice dimensions of equipment manufacturing means broadening our understanding of innovation to include those who build and maintain its infrastructure. It requires centering the experiences of workers and communities in manufacturing zones--not just as labor inputs, but as people with rights, needs, and knowledge of their own environments.
A planetary justice approach to this stage of the AI supply chain calls for more than ethical sourcing audits, or cleaner production lines. It asks how production can be organized to respect workers’ health and autonomy, how environmental safeguards can be co-developed with local communities, and how technological development can be shaped by inclusive, cross-border dialogue rather than imposed through design pipelines based in global tech capitals.
At the AI + Planetary Justice Alliance, we explore how justice can be embedded not just in AI’s outputs, but in its origins--in the physical systems and human labor that make AI possible. This means rethinking what it means to “build AI responsibly,” and who gets to define that responsibility.