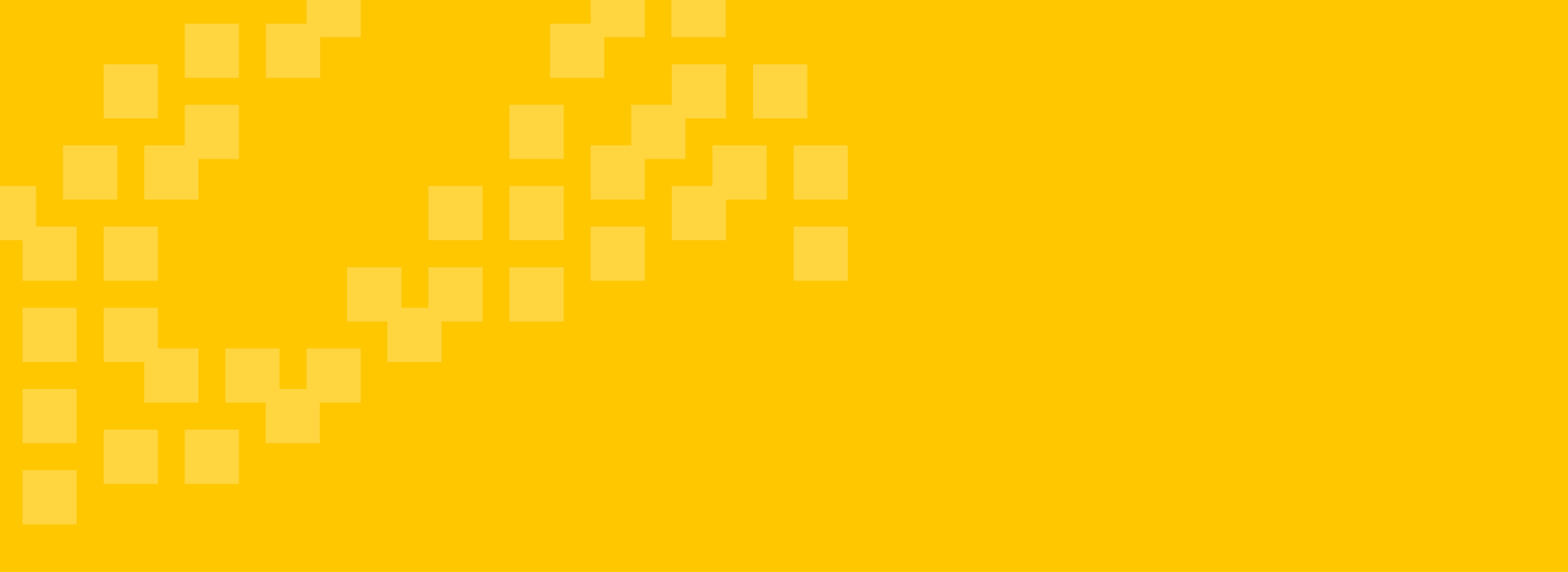
Disposa/End-of-life
What Happens When AI Systems Wear Out?
After an AI system has been trained, deployed, and integrated into the world, its story does not end. Like all technologies, AI has a physical footprint—one that persists and accumulates long after its computational work is done. The final stage of the AI supply chain is often the least visible: the disposal, repurposing, or abandonment of its material components.
This includes the servers, GPUs, cooling systems, sensors, cables, and devices that enable AI to function. As models are upgraded and hardware is replaced, older infrastructure is often discarded, exported, or stockpiled. Much of it becomes part of the global flow of electronic waste (e-waste)—an increasingly urgent environmental and social issue.
-
Decommissioning and Downgrading
As AI systems evolve, so too does the hardware that supports them—from data center servers and GPUs to embedded sensors, smartphones, edge devices, and autonomous systems. Some are sold into secondary markets or repurposed for less demanding tasks. Others are sent to recycling centers or disposed of entirely. These decisions are often made with cost and logistical ease in mind, not environmental impact.Global E-Waste Trade
Many obsolete components are shipped abroad—often to regions with less stringent environmental regulation. Countries in West Africa and Southeast Asia receive large volumes of e-waste, sometimes under the label of "used electronics." Once there, disposal and recycling often take place in the informal sector, with minimal worker protection or environmental safeguards.Landfilling, Burning, or Leaching
When hardware is not responsibly recycled, it is frequently landfilled, burned, or left to degrade in open-air dumps. Toxic substances—such as mercury, lead, cadmium, and brominated flame retardants—can leach into soils and water, or become airborne pollutants. These impacts linger far beyond the lifespan of the AI models they once served.Software Degradation and Digital Obsolescence
End-of-life also includes the software side. As systems evolve, older AI models may become incompatible with new platforms, unsupported by updates, or simply fall out of use. When tools are no longer maintained, questions emerge about data stewardship, long-term accountability, and the potential risks of "orphaned" systems.
-
The end-of-life stage may appear distant from AI development or deployment, but its consequences are deeply embedded in global systems of waste, inequality, and environmental degradation. Disposal is not a passive process—it is structured by decisions about which materials are used, how long products are designed to last, and who is left to deal with their afterlives.
Much of AI’s physical infrastructure is built without long-term end-of-life planning. Short upgrade cycles and planned obsolescence drive demand for newer hardware, while insufficient incentives for repair, reuse, or circular design lead to premature disposal. Meanwhile, the social and ecological impacts of e-waste are concentrated in places that are structurally marginalized from the decision-making processes that shape AI’s growth.
The materials in AI devices—cobalt, rare earths, lithium—are not only resource-intensive to extract, but also difficult to safely recover. Current recycling systems capture only a fraction of these materials, and informal e-waste economies expose workers to hazardous conditions for minimal economic return.
The consequences of disposal are borne not just by people, but by ecosystems: polluted waterways, soil degradation, and wildlife exposure to heavy metals. The AI lifecycle doesn’t simply end—it disperses, quietly embedding itself in landscapes and bodies long after the original innovation cycle has moved on.
-
Toxic exposure → In communities near e-waste processing hubs—particularly where dismantling occurs informally or with minimal regulation—exposure to hazardous substances is a slow and cumulative risk. Toxicants such as lead, mercury, cadmium, and brominated flame retardants can leach into soil and groundwater, or become airborne as particulate matter. Children, pregnant individuals, and informal workers often face disproportionate health risks, not as isolated incidents but as part of broader patterns of environmental injustice.
Labor precarity and informal economies → Much of the labor involved in breaking down and extracting value from discarded AI infrastructure is carried out in informal economies, often under unsafe and insecure conditions. Workers in places like Agbogbloshie (Ghana), Moradabad (India), and Guiyu (China) perform critical tasks—disassembling devices, recovering metals, and sorting components—yet typically without formal protections, fair compensation, or decision-making power. While these activities support livelihoods, they do so under conditions shaped by structural inequality, not equitable choice.
Ecological disruption and more-than-human harm → The end-of-life phase of AI has significant consequences for local ecologies and nonhuman beings. Open burning, acid leaching, and improper landfill disposal contaminate the habitats of fish, birds, insects, and terrestrial animals. Polluted waterways can alter aquatic ecosystems for generations, while soil toxicity affects crop viability and microbial life. These harms often go unnoticed in standard assessments of AI impact, but from a planetary justice perspective, they are central: ecosystems and more-than-human beings also bear the cost of technological discard.
Geographies of discard → The movement of obsolete hardware across borders—often from wealthier nations to those with fewer environmental protections—follows longstanding patterns of economic and geopolitical asymmetry. While e-waste may be shipped under the banner of reuse or circularity, in practice it frequently arrives in conditions that lack the infrastructure for safe processing or ethical disposal. This global geography of discard reproduces what has been called waste colonialism, where some regions become enduring endpoints for the toxic legacies of digital systems developed elsewhere.
-
The end-of-life phase of AI hardware—including servers, sensors, and edge devices—reveals significant geopolitical disparities in how e-waste is managed. While the economic value generated by AI technologies is predominantly captured by corporations and consumers in the Minority World, the environmental and health burdens associated with e-waste disposal are often externalized to the Majority World.
Countries such as China, India, Ghana, and Nigeria have become primary destinations for global e-waste. In these regions, informal recycling sectors handle the majority of e-waste processing, frequently employing hazardous methods like open-air burning of cables to extract copper and acid baths (e.g., nitric/hydrochloric acid) to recover gold and other metals, releasing toxic fumes. These practices pose serious health risks to workers and contribute to environmental degradation. For instance, Guiyu in China has been identified as one of the largest e-waste processing sites globally, where improper handling has led to significant pollution and health issues among local populations.
Despite international agreements like the Basel Convention (1992), which aims to regulate the transboundary movements of hazardous wastes, enforcement challenges persist. Loopholes and limited regulatory oversight allow substantial amounts of e-waste to be exported from developed to developing countries under the guise of recycling or second-hand use. This ongoing practice underscores a form of "digital dumping," where the environmental costs of technological advancement are disproportionately borne by less affluent nations.
Addressing these inequities requires a comprehensive approach that includes stronger international enforcement mechanisms, investment in safe recycling infrastructure within developing countries, and corporate responsibility initiatives that prioritize ethical end-of-life management of AI hardware.
-
A planetary justice-centered approach to AI’s end-of-life invites us to recognize that disposal is not an afterthought or a neutral endpoint. It is a continuation—and often a concentration—of the same patterns of extraction, externalization, and asymmetry that structure earlier stages of AI’s lifecycle. What is left behind when AI systems are no longer useful can reveal as much about how they were built as what they were built for.
Reducing harm at this stage requires a rethinking of design logics, market incentives, and material cultures. Designing AI hardware for repair, reuse, and disassembly–rather than planned obsolescence–is a crucial step, but so too is building the global infrastructure and social conditions that make such practices viable, equitable, and non-extractive. This includes supporting local repair economies, strengthening labor protections in recycling sectors, and ensuring that transitions to “circularity” do not become new sites of dispossession or greenwashing.
At the same time, a planetary justice lens asks us to interrogate the broader systems that drive premature obsolescence. Are the benefits of faster, more powerful models sufficient to justify the environmental and social costs of accelerated hardware turnover? Who determines what counts as an upgrade, and who bears the weight of the systems that are left behind?
These questions extend beyond the human. Disposal affects not only workers and communities, but ecosystems and more-than-human worlds. The slow release of toxins into soil and water, the degradation of landscapes, and the disruption of ecological relationships all speak to a need for ethics that include nonhuman life—not as background, but as co-inhabitants of the environments AI systems touch.
From this perspective, the end-of-life phase is not simply about managing waste—it becomes an opportunity to ask: What does it mean to let go of a digital technology well? What practices of care, accountability, and repair are possible when a system stops working or is no longer needed? And how might those practices shape ifand how we build in the first place?